Publish an
GenAI-powered app
with a
no-code platform
that integrates seamless with OpenAI.
Unlocking the Potential of RAG GenAI: The Future of Intelligent Content Generation
What is RAG GenAI?
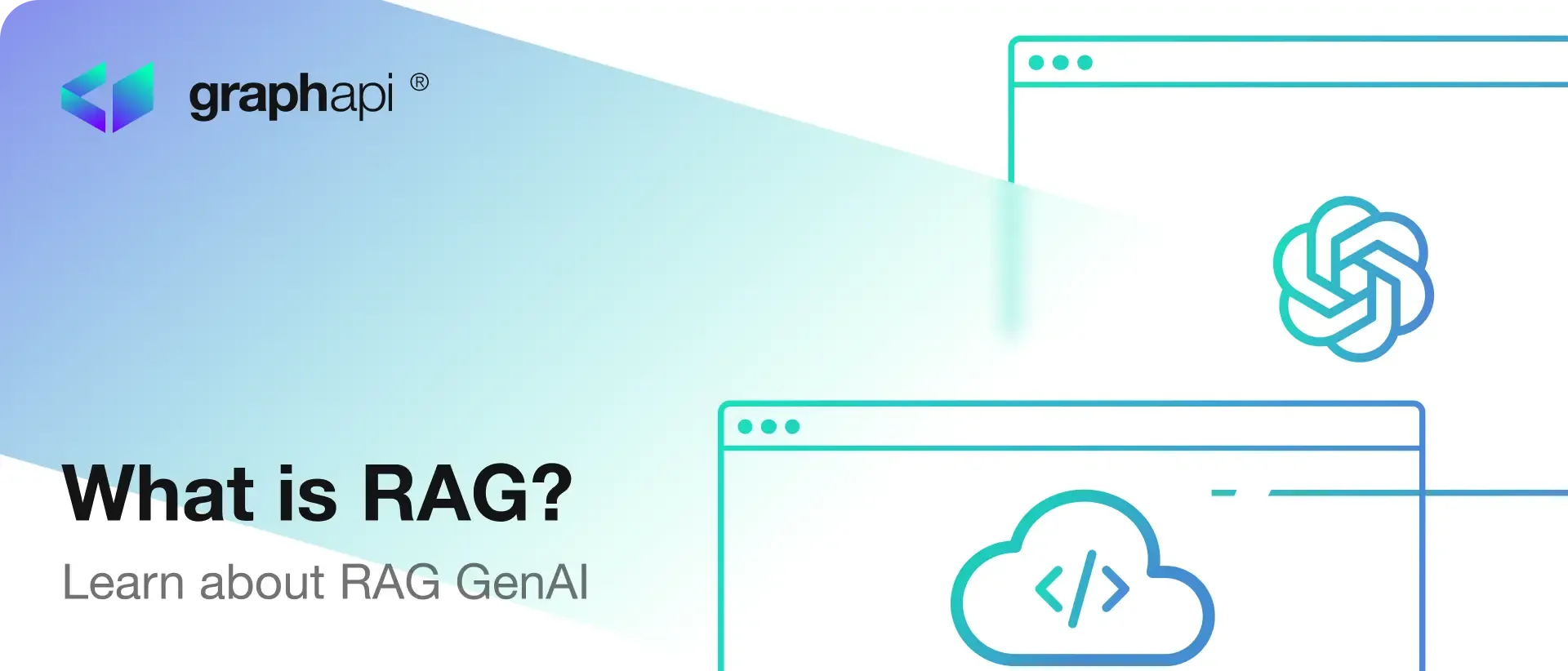
The world of artificial intelligence continues to evolve, introducing cutting-edge technologies that enhance how we interact with information and solve complex problems. One such advancement is RAG GenAI or Retrieval-Augmented Generation in Generative AI. This hybrid approach combines the power of information retrieval with generative models to create highly accurate, context-aware outputs. Let's dive into what RAG GenAI is and how it delivers enhanced performance by merging retrieval and generation.
Definition of RAG (Retrieval-Augmented Generation) in Generative AI
At its core, RAG is an AI architecture that integrates retrieval mechanisms with generative models to improve the quality, relevance, and accuracy of responses generated by AI systems. Traditional generative AI models, like GPT, rely solely on pre-trained datasets. Large Language Models (LLMs) are powerful; however, they sometimes produce outdated or incorrect information due to the static nature of their training data. LLMs have limited context windows, which amplifies the problem even more.
RAG addresses this limitation by incorporating an active retrieval layer. Here's how it works:
- The retrieval component searches external databases, documents, or live information sources to gather relevant context in real time.
- The generative component inputs this retrieved information to create accurate, contextually relevant responses.
By augmenting the generative process with retrieval, RAG enables AI systems to produce answers grounded in the most up-to-date and specific information, making it ideal for applications requiring factual accuracy.
How RAG GenAI Combines Retrieval and Generation for Enhanced Performance
The key innovation in RAG GenAI lies in its ability to blend two traditionally separate AI functions: retrieval and generation.
This combination enhances the system's performance in several ways:
- Contextual Accuracy: RAG leverages real-time retrieval from external knowledge bases to ensure the generated output is both relevant and accurate. For example, a RAG-powered chatbot can retrieve the latest product information or policy updates to provide precise answers in customer support.
- Reduced Hallucination in AI Outputs: Generative models can sometimes produce "hallucinations"—confidently presented but incorrect information. RAG minimizes these inaccuracies by grounding the output in retrieved data and delivering trustworthy responses.
- Dynamic Knowledge Updates: Unlike static models, RAG dynamically integrates new information during retrieval, ensuring the system remains current without requiring extensive re-training.
- Improved Scalability: The retrieval layer can access large-scale knowledge repositories, enabling the AI to tackle complex queries that would overwhelm traditional generative systems.
- Enhanced User Experience: RAG delivers highly informative and conversationally engaging responses by combining precise retrieval with natural language generation, offering a seamless user experience.
How RAG GenAI Works
Retrieval-augmented generation (RAG) combines the strengths of knowledge retrieval and generative AI models. This integration allows RAG GenAI to provide accurate, context-rich, and highly relevant responses to complex queries. Here's a closer look at how RAG GenAI operates and the components that make it so effective.
The Role of Knowledge Retrieval in a RAG Stack
Knowledge retrieval forms the backbone of RAG's ability to deliver precise and up-to-date information. Generative models, like LLMs, rely solely on pre-trained data; RAG, however, incorporates a retrieval layer that actively searches external data sources in real time, ensuring the AI system can access the most current and contextually relevant information.
Key aspects of the retrieval process include:
Dynamic Querying: RAG generates a query based on the user's input and sends it to an external knowledge base, such as a database, API, or document repository.
Contextual Matching: The retrieval system identifies and extracts the most relevant information based on semantic similarity and context.
Real-Time Updates: By accessing live data sources, RAG ensures that its outputs reflect the latest information, reducing the risk of outdated or inaccurate responses.
For example, in customer support scenarios, RAG can retrieve details about recent product updates or policy changes, ensuring that users receive accurate answers tailored to their needs.
Generative Models and Their Contribution to RAG
The generative component of RAG is responsible for crafting natural, coherent, and conversational responses using the information retrieved by the system. These models, such as ChatGPT, are pre-trained on large datasets and excel at processing natural language.
Interpreting Context
Generative models analyze the retrieved data alongside the user's query to produce contextually relevant responses.
Enhancing Clarity
They translate raw data into human-readable language, ensuring the end user quickly understands the output.
Providing Personalization
Generative models adapt their tone and style to align with the user's intent, making interactions more engaging and user-friendly.
RAG minimizes errors and delivers informative and conversationally smooth outputs by combining retrieval with generation.
Integration of Retrieval and Generation Processes
The true power of RAG lies in the seamless integration of retrieval and generation processes. This synergy enables the system to produce outputs grounded in factual data while maintaining the flexibility and fluency of generative AI.
Key elements of this integration include:
Pipeline Architecture
The user's query flows through the retrieval layer, where relevant data is identified and extracted. This data is then passed to the generative layer to craft the final response.
Feedback Loops
RAG systems often include iterative mechanisms that refine the retrieval process based on the generated output, ensuring continuous improvement.
Dynamic Adaptability
The system dynamically adjusts its retrieval and generation strategies based on the complexity of the query, optimizing performance for a wide range of use cases.
For instance, in a legal research application, RAG can retrieve specific clauses from legal documents and present them as part of a coherent explanation, enabling users to quickly grasp critical information without sifting through extensive texts.
Benefits of Using RAG GenAI
In the ever-evolving world of artificial intelligence, Retrieval-Augmented Generation (RAG) GenAI stands out as a transformative technology. RAG delivers unparalleled accuracy, speed, and scalability benefits by combining dynamic data retrieval with generative AI capabilities. Let's explore the key advantages of using RAG GenAI.
Improved Accuracy Through Contextual Knowledge
One of the most significant benefits of RAG GenAI is its ability to enhance accuracy by grounding responses in real-time, contextually relevant data. Unlike traditional generative models that rely solely on static training datasets, RAG incorporates a retrieval layer to access external knowledge bases dynamically.
By sourcing information directly from reliable and up-to-date databases, RAG minimizes errors and "hallucinations" in its outputs.
RAG tailors its responses to the specific context of a query, ensuring that answers are precise and aligned with user intent.
The retrieval mechanism allows RAG to stay current with the latest information, making it ideal for industries like healthcare, finance, and customer support.
For instance, in a medical application, RAG can retrieve and combine the latest research findings with its generative capabilities to provide accurate and detailed explanations.
Faster Response Times for Complex Queries
RAG GenAI delivers rapid responses, even for intricate or multifaceted queries. By integrating retrieval and generation, the system streamlines complex information processing.
Optimized Query Handling
The retrieval layer quickly identifies relevant data, reducing the computational load on the generative model.
Efficiency in Processing
RAG minimizes the need for exhaustive searches or extensive pre-computed datasets, enabling faster response generation.
Enhanced User Experience
Faster response times translate to smoother and more efficient interactions, which is especially crucial in time-sensitive applications like customer support or legal research.
For example, a legal advisor powered by RAG GenAI can instantly retrieve and summarize relevant case laws, saving professionals hours of manual research.
Scalable Solutions for Data-Driven Applications
As businesses increasingly rely on data-driven decision-making, RAG GenAI's scalability makes it a powerful tool for handling large volumes of information across various domains.
- Adaptable Architecture: RAG systems can seamlessly scale to accommodate growing datasets and evolving user needs.
- Cost Efficiency: By leveraging dynamic retrieval, RAG reduces the need for extensive re-training of generative models, lowering operational costs.
- Versatility Across Industries: Whether e-commerce, education, or enterprise analytics, RAG's scalable design allows it to adapt to diverse use cases.
RAG GenAI can analyze massive product catalogs and customer queries in retail to provide personalized recommendations at scale, enhancing customer satisfaction and driving sales.
Challenges in Implementing RAG GenAI
While Retrieval-Augmented Generation (RAG) GenAI offers groundbreaking advantages in artificial intelligence, implementing it is challenging. Organizations must navigate several complexities, from data quality issues to ethical concerns, to fully leverage their potential. Here, we delve into the key challenges in deploying RAG GenAI systems.
Data Quality and Retrieval Accuracy
The foundation of RAG GenAI's effectiveness lies in the quality of the data it retrieves. Poor data quality or inaccurate retrieval can undermine the system's performance.
The generated outputs will reflect these inaccuracies if the external knowledge base contains incomplete or outdated information.
Multiple conflicting sources can confuse retrieval, leading to inconsistent or irrelevant responses.
Ensuring that the retrieval mechanism understands and matches the user's query accurately requires robust natural language processing capabilities.
To address these issues, organizations must invest in maintaining clean, up-to-date data repositories and fine-tuning retrieval algorithms for precision.
Balancing Performance and Computational Costs
RAG GenAI systems integrate retrieval and generation processes, which can place significant demands on computational resources.
High Resource Consumption
The retrieval layer requires real-time querying of large datasets, while the generative layer processes this information to create outputs. This dual-layer operation can strain system performance.
Latency Challenges
Real-time data retrieval can introduce delays, particularly when accessing large or distributed databases.
Cost of Scalability
Scaling RAG systems to handle increased data volume and user demand can escalate infrastructure and operational costs.
Optimizing system architecture, employing caching strategies, and leveraging cloud-based solutions can help mitigate these challenges and enable efficient performance at scale.
Ethical Considerations in Knowledge Retrieval
The retrieval aspect of RAG GenAI raises several ethical concerns that operators must carefully manage to ensure the technology's responsible use.
- Bias in Retrieved Data: The outputs may perpetuate or amplify biases if the knowledge base contains biased or unverified information.
- Privacy and Security Risks: Retrieving data from sensitive or restricted sources can lead to privacy violations or unauthorized access.
- Transparency and Accountability: To maintain trust and accountability, users must understand how the system retrieves and uses data.
To navigate these ethical challenges, organizations should prioritize data transparency, implement robust privacy safeguards, and conduct regular audits to identify and address potential biases.
The Future of RAG GenAI
As artificial intelligence advances, Retrieval-Augmented Generation (RAG) GenAI plays a pivotal role in shaping the next generation of AI applications. By combining the strengths of retrieval and generative capabilities, RAG offers unparalleled opportunities for innovation across industries. Let's explore the future of RAG GenAI, focusing on emerging trends and the growing importance of hybrid AI models.
Emerging Trends and Innovations in RAG Technology
As breakthroughs in both retrieval and generative technologies drive the evolution of RAG GenAI, we see the following key trends emerging:
Real-Time Data Integration
Future RAG systems will seamlessly integrate with live data streams, enabling AI to provide real-time, contextually relevant responses in dynamic environments such as financial markets, e-commerce, and customer support.
Domain-Specific Fine-Tuning
As industries adopt RAG, the emphasis will be on creating domain-specific knowledge bases and tuning models to deliver highly specialized insights tailored to unique business needs.
AI-Driven Retrieval Enhancements
Advances in natural language processing and semantic search algorithms will make the retrieval layer more accurate, reducing errors and improving the quality of generated content.
Multimodal Capabilities
RAG systems will expand beyond text to include images, videos, and other data types, opening up new possibilities for healthcare, education, and entertainment applications.
Edge Computing Integration
Future AI systems will also leverage edge computing to process data locally, addressing latency and scalability challenges and reducing their reliance on centralized servers.
These innovations will make RAG GenAI more powerful, versatile, and accessible, solidifying its role as a cornerstone technology in the AI landscape.
The Growing Importance of Hybrid AI Models
RAG GenAI exemplifies the power of hybrid AI models that combine the best of two worlds: the precision of retrieval mechanisms and the creativity of generative AI. Businesses expect AI systems to deliver accuracy and adaptability, so this hybrid approach is becoming increasingly important.
Hybrid models like RAG provide users with reliable, data-driven insights while maintaining the flexibility to address ambiguous or open-ended queries. By integrating retrieval and generation, hybrid models can tackle complex tasks such as legal research, scientific discovery, and personalized marketing, which demand factual accuracy and contextual understanding.
Hybrid models optimize resource usage by focusing computational power where it's most needed—retrieving relevant data or generating tailored responses—making them ideal for large-scale applications.
The dual-layer approach of RAG allows users to trace the origins of information in AI-generated outputs, fostering greater trust and transparency in AI systems.
As organizations demand AI solutions that balance innovation with reliability, the importance of hybrid models like RAG GenAI will only continue to grow. These models represent the future of AI, where precision meets creativity to solve the most pressing challenges.